Historic Digital Survey (HDS) is a research project that is looking into the ways digital documentation and data processing can benefit the maintenance and repair of the traditional built environment. The project, conducted by the University of Edinburgh and Heriot-Watt University in collaboration with Historic Environment Scotland, aims to make use of point-cloud data to enable better monitoring, diagnosis, maintenance and repair.
Traditional visual approaches to condition surveys are time-consuming and still often include incorrect labelling, misdiagnosis and omission of defects. This is caused because surveyors differ in the way they report which is linked to their experience – or sometimes inexperience – and the compressed time frames for completing surveys. Providing accurate surveys is vitally important, though, as incorrect identification and diagnosis of defects can result in inappropriate interventions that ultimately undermine the fundamental conservation objectives they mean to support.
"Traditional visual approaches to condition surveys are time-consuming and still often include incorrect labelling, misdiagnosis and omission of defects"
In this context, progressive implementation of digital documentation and subsequent application of innovative data processing tools such as machine learning (ML) algorithms could transform surveying, repair and maintenance.
Digital documentation in the form of laser scanning and photogrammetry along with bespoke, supervised ML algorithms could, for instance, benefit ashlar masonry defect detection. Masonry constitutes a significant proportion of the international historic built environment. In 2018, Historic Environment Scotland estimated there are 500,000 pre-1919 traditional – or historic – buildings in Scotland, ostensibly constructed in masonry and lime-based materials. Repair and maintenance expenditure for such a vast amount of buildings is therefore high.
Although there are many forms of masonry, ashlar is one of the most prominent around the world, associated with high-status architecture reflecting the time and cost associated with its construction. It is characterised as regular, square masonry with tight mortar joints of around 3mm and fine-tooled or polished visible faces.
Surveying historic ashlar walls effectively and efficiently is very time-consuming given the number of individual masonry units and the need to label their individual condition manually. Furthermore, these surveys are prone to error as the identification and labelling of condition and decay mechanism can be complex, reflecting the variability in materials exposure and build quality.
Stirling Castle chapel
As part of the HDS project, a digital approach was tested on the main facade of the Chapel Royal at Stirling Castle. The castle typifies ashlar masonry construction and experiences many of the defects most commonly encountered in natural stone by surveyors, such as erosion and discolouration due to salts or moisture.
The chapel, maintained by Historic Environment Scotland, was built in 1594. It is a category A listed building and a scheduled ancient monument. The architectural fenestration of the facade is characterised by a central doorway, flanked by coupled columns, and three Romanesque arched mullioned windows to either side of the entrance.
The first stage of the survey used digital documentation technologies to acquire data on the geometry and colour of the chapel facade. Several laser scans were taken at approximately 10m from the wall, and a set of photographs taken to produce a 3D point cloud of the scene through structure-from-motion photogrammetric techniques.
Both point clouds, obtained from terrestrial laser scanning (TLS) and photogrammetry, were registered in the same coordinate system. Colour information in the TLS cloud was then automatically replaced with RGB data from the photogrammetric cloud, as colour from digital single-lens-reflex cameras is of higher quality than that captured by the TLS device.
As a result, the point cloud is characterised by high-quality geometric data from TLS and high-quality colour data from photogrammetry. This point cloud was then automatically segmented into the individual ashlar units using a technique based on the approach detailed on p.31 of the May/June 2017 edition of the Building Conservation Journal. The approach has been implemented as free open-source software.
In parallel with this activity, professionals with expertise in stone defects carried out a comprehensive survey. This was reported in a context of stone deterioration patterns in an attempt to attain a common understanding of defects that were present. The survey adopted terminology outlined in ICOMOS-ISCS: Illustrated glossary on stone deterioration patterns. During the surveying process, primary masonry defects were identified, including erosion, delamination, discoloration and mechanical damage. In broad terms, these defects reflect the identification of loss and gain of material and colour change. Some 500 masonry units were manually identified and labelled on site, and 650 individual labelled defects were assigned by means of a tailored graphic user interface.
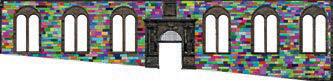
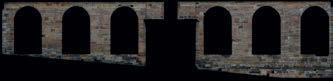
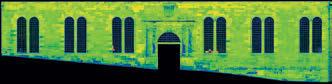
The labelled defective regions were used as training data for the ML algorithm. Critical to any supervised ML algorithm is its reliance on such inputs, which are fed in to yield meaningful and accurate labelled outputs, which should correspond to the surveyors' observations. As training data is only as good as the individual identifying and creating the inputs, expert surveyors were hired to collaborate on decisions.
Finally, the trained ML algorithm, based on a logistic regression model, was employed to survey the fabric, and decayed areas affected by both colour- or geometry-related deteriorations were detected and automatically classified. The major defect categories present in the surveyed facade were erosion, mechanical damage and discolouration. The algorithm classified erosion patterns in areas with high moisture load – for example at the base of walls – and areas of high run-off where there are defective rainwater goods and detailing. In addition, discolouration was associated with salts prevalent where the masonry dries at the base of the wall. Interestingly, the mechanical damage distribution was associated with gunshots, because the palace was at one time used as barracks.
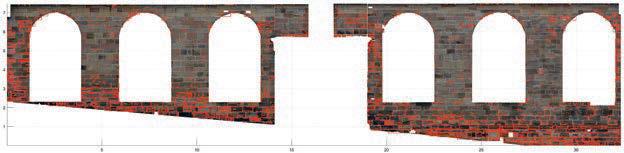
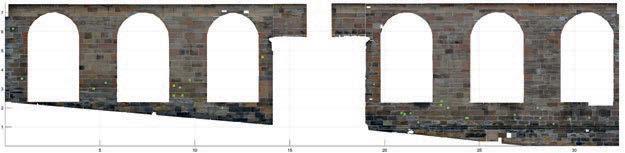
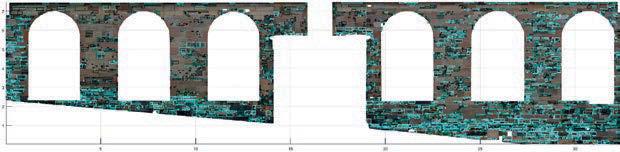
The algorithm achieved an accuracy of 93 per cent in comparison to our meticulous manual survey conducted by experts. Its ability to identify specific areas of interest or defect types helps our understanding of defect distribution, and therefore diagnosis.
"The repeatability of these digital surveys could herald a paradigm shift in our capacity to monitor change in historic building fabric in an interoperable manner"
While the approach used at Stirling offers promising results, we must nonetheless highlight that the effective application of statistical models remains challenging, particularly because algorithms have to perform well in a general sense and not just in individual cases. It is clear that more data is required to test the algorithm, but that it will improve over time.
The repeatability of these digital surveys could also herald a paradigm shift in our capacity to monitor change in historic building fabric in an interoperable manner. In addition, the progressive use of data in point clouds will be more pronounced as historic BIM is used more widely.
The impact of artificial intelligence on work, a 2018 survey by the Royal Society and the British Academy, reflects a fear of jobs losses; however, the aim of using digital technologies in the built environment is not to replace the surveyor but to redirect their higher-order skills to add value to their work. This will move surveyors away from relatively mundane operations and redirect funds from surveying towards fabric repair and maintenance intervention.
Dr Alan Forster is associate professor in building conservation low-carbon materials and construction technology in the School of Energy Geoscience Infrastructure and Society at Heriot-Watt University a.m.forster@hw.ac.uk
Dr Enrique Valero is a research associate in the Institute of Infrastructure and Environment and Dr Frédéric Bosché is senior lecturer in infrastructure programme management at the University of Edinburgh
e.valero@ed.ac.uk f.bosche@ed.ac.uk
Dr Ewan Hyslop is head of technical research and science Lyn Wilson is a digital documentation manager and Aurélie Turmel is conservation science manager at Historic Environment Scotland
ewan.hyslop@hw.ac.uk lyn.wilson@hes.scot aurelie.turmel@hes.scot
Related competencies include: BIM management
Building conservation accreditation competencies include: Diagnosis of defects